Materials Discovery
The materials discovery project is a collaboration between the Institute for Computational Sustainability (ICS), the van Dover Group (Materials Science & Engineering Department), the Energy Materials Center at Cornell (emc²), and the Joint Center for Artificial Photosynthesis (JCAP).
This is contributing to a larger effort in high-throughput materials science, which is part of the Materials Genome Initiative (MGI).
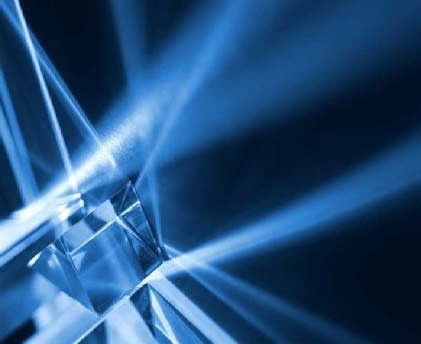
Credit: NIST
Better Materials for Energy Devices
This project aims to develop new materials for more environmentally friendly, sustainable, and economical fuel cells, batteries, solar fuel generation, etc.
Crystal Structure-Function Relationship
The process is to identify and chemical elements likely to form compounds with desired properties, synthesize a range of mixtures as a thin-film powder on a glass or silicon substrate, screen for suitability, and identify the crystal structure of selected materials.
Structure from X-ray Crystallography
When an X-ray beam goes through a crystal, the regular spacing of atoms causes bright spots to appear on a detector. When a powder contains millions of crystals, these bright spots can appear as rings.
The resulting patterns are characteristic of the underlying crystal structure. We measure X-ray diffraction (XRD) patterns at hundreds of different mixture compositions, in order to learn how structure changes with composition.
Crystalline Phases
In this context, a phase is a set of chemical compounds with similar composition, function and structure. Each of the diffraction patterns we measure can be a mixture of different types of crystals, or phases.
Source Separation Problem
The problem is to find out at the same time which phases were formed, and how and where in the powder mixture they appear. This is an example of a source separation problem.
Visual Pattern Recognition
The phase map identification problem turns out to be difficult to solve computationally. The data contain patterns that, once found, can help to find the answer. When visualized in certain ways, these patterns are often easy for people to recognize.
So our approach is to have humans quickly identify patterns in small subsets of the data, and use this information to guide a more complete automatic solver.